From ZDNet:
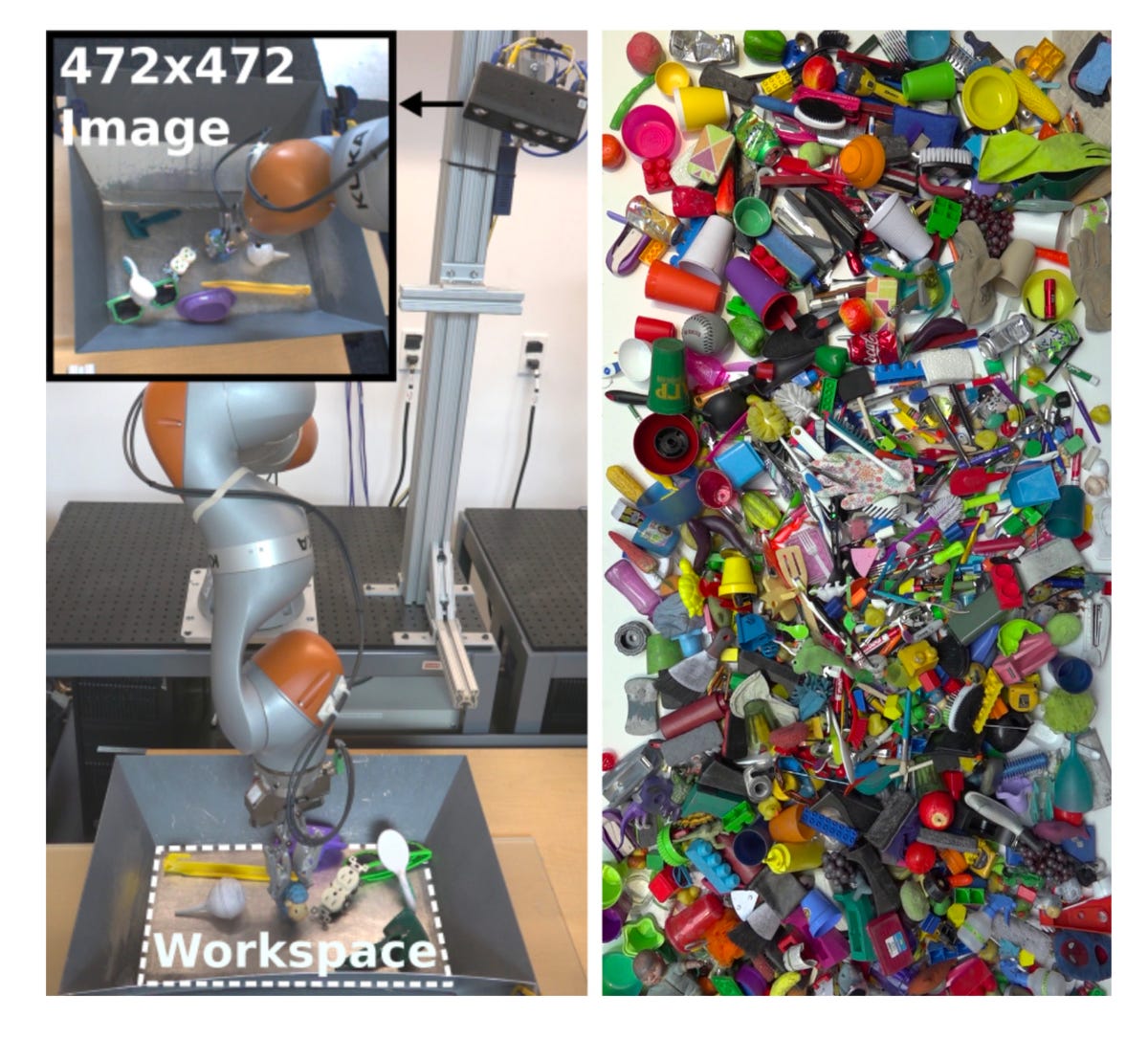
"one of the most significant open problems in robotics," according to Sergey Levine and collaborators.
Grasping is a good example of problems that bedevil real-world machine learning, including latency
that throws off the expected order of events, and goals that may be difficult to specify.
The vast majority of artificial intelligence has been developed in an idealized environment: a computer simulation that dodges the bumps of the real world. Be it DeepMind's MuZero program for Go and chess and Atari or OpenAI's GPT-3 for language generation, the most sophisticated deep learning programs have all benefitted from a pruned set of constraints by which software is improved.
For that reason, the hardest and perhaps the most promising work of deep learning may lie in the realm of robotics, where the real world introduces constraints that cannot be fully anticipated.
That is one takeaway from a recent report by researchers at the University of California at Berkeley and Google who have summarized several years of experiments with robots using what's known as reinforcement learning....
....MUCH MORE
Also at ZDNet
Google’s deep learning finds a critical path in AI chips
Google is betting the company on AI and if what they are working on pans out they will be doing stuff that is literally beyond comprehension.