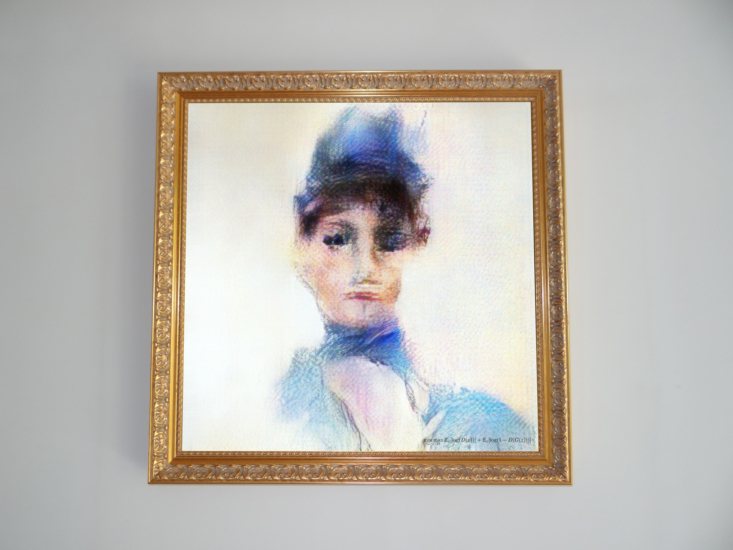
Image: Madame De Belamy, from the collection La Famille de Belamy by the group Obvious.
Recently, a work of AI-generated art, a portrait called Edmond de Belamy, was auctioned for $432,500. It was based on code written by Robbie Barrat, a researcher who didn’t see any of that money. As this Verge article by James Vincent details, Barrat’s code was adapted by a group of French students who managed to get the art world to pay them for what it produced. In a sense, the artwork was this performance: not the AI-generated image but the way it found itself into a Christie’s auction and a lot of media coverage. The image itself — a fairly unremarkable portrait; not one of those disturbing Google Deep Dream images with eyeballs and dog faces emerging from plates of spaghetti — is more like residual documentation.
Work like Barrat’s and that of Janelle Shane — a researcher who writes the AI Weirdness blog — puts a friendly face on machine learning, highlighting its generative fun side: how you can use neural nets to make improbable Halloween costumes or designer clothes, or to play flarf-style language games. In an interview with Arabelle Sicardi, Barrat says, “working with AI and generative art is nice because people can’t really misuse your software or your results.” (Which seems like a generous thing to say when your work has been hijacked and auctioned off.)
I find this kind of work irresistible. I like how I can feel surprised by it, how I read it as unmotivated. It never comes across as trying too hard; instead I can adopt a kind of patronizing attitude toward the machines. Aren’t they cute? The way the systems “learn,” often staged in write-ups of these projects as a series of clumsy steps toward coherence, comes across as a kind of serendipity, an accidental teleology. It’s not aesthetic purposefulness per se but some kind of deeper destiny being put on display.
Of course, human intention is still driving these projects, but it is abstracted a step away from the output. Barrat suggests that AI can “augment artists’ creativity” by producing “surreal” combinations that the artist can then sift through or refine. “A big part of my role in this collaboration with the machine is really that of a curator, because I’m curating the input data that it gets, and then I’m curating the output data that the network gives me and choosing which results to keep, and which to discard.” He can adjust the data sets and parameters until the output is suitably familiar or surprising or some surreal blend of both. Sicardi suggests that the machine can overcome pockets of resistance in the artist’s mind: “When you actually put an algorithm in your hands, it forces you to create versions and derivatives. It draws conclusions you wouldn’t have considered, because it lacks the context that may inhibit you.” AI programmers are then in the paradoxical position of producing intentional accidents — works that reflect their sensibility or their sense of rightness without their having to directly create them.
Moreover, they feel right because they surprise the artist/researcher with their fittingness even as they continue to seem like they just happened. The works thereby embody a sense of plausible disavowal: It was what I was going for but not really, the machines took it somewhere no one could expect.
Algorithms generally are deployed for disavowal: as if they could eliminate bias or at least distract from it. They obfuscate the human input into a particular decision-making process to make it appear more objective. This typically means that the source of bias is displaced into the data — what was chosen to be collected and fed to the algorithms, and what assumptions have governed the programmer’s coding. Algorithmic processing and machine learning can make it appear as though the systems decide for their own reasons, reproducing the biases of the past as if no one is responsible for them, as if they are inherent. This, in the view of AI researcher Ali Rahimi, makes machine learning into a kind of alchemy.
Vincent attributes the French students’ art world success in part to “their willingness to embrace a particular narrative about AI art, one in which they credit the algorithm for creating their work.” This makes it a variant on what Astra Taylor has called “fauxtomation,” and what Jathan Sadowski described as Potemkin AI — where production processes are represented as artificial intelligence to devalue the human labor that is actually doing the work. But here, the undue attribution to AI scrambles the way we understand not how the artwork was produced but what its commodification confers.
If AI made the image, auctioning it off is not an unfortunate commercialization of human aesthetic creativity but the act that breathes “art” into something otherwise merely machinic. The work’s aesthetic value becomes equivalent to its monetary value — the image is significant only because someone paid something for it particularly, and that price is effectively its “content.” With algorithms sidelining artists, Christie’s can take centerstage, as Vincent points out: The auctioneers, he reports, have “presented the auction as a provocative gesture that refreshes the company’s brand and stakes its claim in any lucrative new art market.”
When AI art is treated as if it were made by machines rather than researchers, it treats the displacement in agency as an aesthetic, which Christie’s then puts a price to. The pretense of machinic creativity extends the algorithmic alibi, gives it a tangibility: You see? AI really can think on its own, in its own way, and here’s the visual proof. The uncanny works — recognizable as representing something but also appearing vague, alien, inorganic — help reinforce the idea that AI “thinking” is original and not merely derived from the data sets the adversarial networks are trained on, which effectively establish the limits on what can be imagined....MUCH MORE