In the early 1900s, Wilhelm von Osten, a German horse trainer and mathematician, told the world that his horse could do math. For years, Von Osten traveled Germany giving demonstrations of this phenomenon. He would ask his horse, Clever Hans, to compute simple equations. In response, Hans would tap his hoof for the correct answer. Two plus two? Four taps.
But scientists did not believe Hans was as clever as Von Osten claimed. An extensive study, coined the Hans Commission, was conducted by psychologist Carl Stumpf. He found that Clever Hans wasn’t solving equations, but responding to visual cues. Hans would tap up to the correct number, which was usually when his trainer and the crowd broke out in cheers. And then he would stop. When he couldn’t see those expressions, he kept tapping and tapping.
There’s a lot that computer science can learn from Hans today. An accelerating field of research suggests that most of the artificial intelligence we’ve created so far has learned enough to give a correct answer, but without truly understanding the information. And that means it’s easy to deceive.
Machine learning algorithms have quickly become the all-seeing shepherds of the human flock. This software connects us on the internet, monitors our email for spam or malicious content, and will soon drive our cars. To deceive them would be to shift tectonic underpinnings of the internet, and could pose even greater threats for our safety and security in the future.
Small groups of researchers—from Pennsylvania State University to Google to the U.S. military— are devising and defending against potential attacks that could be carried out on artificially intelligent systems. In theories posed in the research, an attacker could change what a driverless car sees. Or, it could activate voice recognition on any phone and make it visit a website with malware, only sounding like white noise to humans. Or let a virus travel through a firewall into a network.

On the left, the unaltered image shows a building. The right
image is altered to be seen as an ostrich by deep neural network-based
image recognition software. The center image shows the slight
distortions being made to the original picture in order to deceive the
algorithm.—Christian Szegedy
Instead of taking the controls of a driverless car, this method shows it a kind of a hallucination—images that aren’t really there.
These attacks use adversarial examples: images, sounds, or potentially text that seems normal to human viewers, but are perceived as something else entirely by machines. Small changes made by attackers can force a deep neural network to draw incorrect conclusions about what it’s being shown.
“Any system that uses machine learning for making security-critical decisions is potentially vulnerable to these kinds of attacks,” said Alex Kantchelian, a researcher at Berkeley University who studies adversarial machine learning attacks.
But knowing about this early on in the development of artificial intelligence also gives researchers the tools to understand how to fix the gaps. Some have already begun to do so, and say their algorithms are actually more efficient because of it.
“We show you a photo that’s clearly a photo of a school bus, and we make you think it’s an ostrich.”
Most mainstream A.I. research today involves deep neural networks, which build on the larger field of machine learning. Machine learning techniques use calculus and statistics to make software we all use, like spam filters in email and Google search. Over the last 20 years, researchers began applying these techniques to a new idea called neural networks, a software structure meant to mimic the human brain. The general idea is to decentralize computing over thousands of little equations (the “neurons”), which take data, process it, and pass it onto another layer of thousands of little equations.
These artificial intelligence algorithms learn the same way machine learning has worked, which is the same way humans learn. They’re shown examples of things and given labels to associate with what they’re shown. Show a computer (or a child) a picture of a cat, say that’s what a cat looks like, and the algorithm will learn what a cat is. To identify different cats, or cats at different angle, the computer needs to see thousands to millions of pictures of cats.
Researchers found that they could attack these systems with purposefully deceptive data, called adversarial examples.

In a 2015 paper,
Google researchers showed it was possible to make deep neural networks
classify this image of a panda as a gibbon, by applying light
distortion.
“We show you a photo that’s clearly a photo of a school bus, and we make you think it’s an ostrich,” says Ian Goodfellow, a researcher at Google who has driven much of the work on adversarial examples.Previously:
By altering the images fed into a deep neural network by just four percent, researchers were able to trick it into misclassifying the image with a success rate of 97 percent. Even when they did not know how the network was processing the images, they could deceive the network with nearly 85 percent accuracy. That latter research, tricking the network without knowing its architecture, is called a black box attack. This is the first documented research of a functional black box attack on a deep learning system, which is important because this is the most likely scenario in the real world.
In the paper, researchers from Pennsylvania State University, Google, and the U.S. Army Research Laboratory actually carried out an attack against a deep neural network that classified images, supported on MetaMind, an online tool for developers. The team built and trained the network they were attacking, but their attacking algorithm operated independent of that architecture. With the attacking algorithm, they were able to force a black-box algorithm to think it was looking at something else with an accuracy up to 84.24 percent....MUCH MORE
Adversarial Images, Or How To Fool Machine Vision
...Interesting that the authors consider fooling facial recognition algos to be an 'attack'....
First though a bit of housekeeping.
Just so you know, I don't actually use the make-up techniques featured in the earlier posts. Despite the fact they have some efficacy at fooling the camera they make you look like a moron to human observers on the street. Better to just put on some glasses and blend into the crowd.
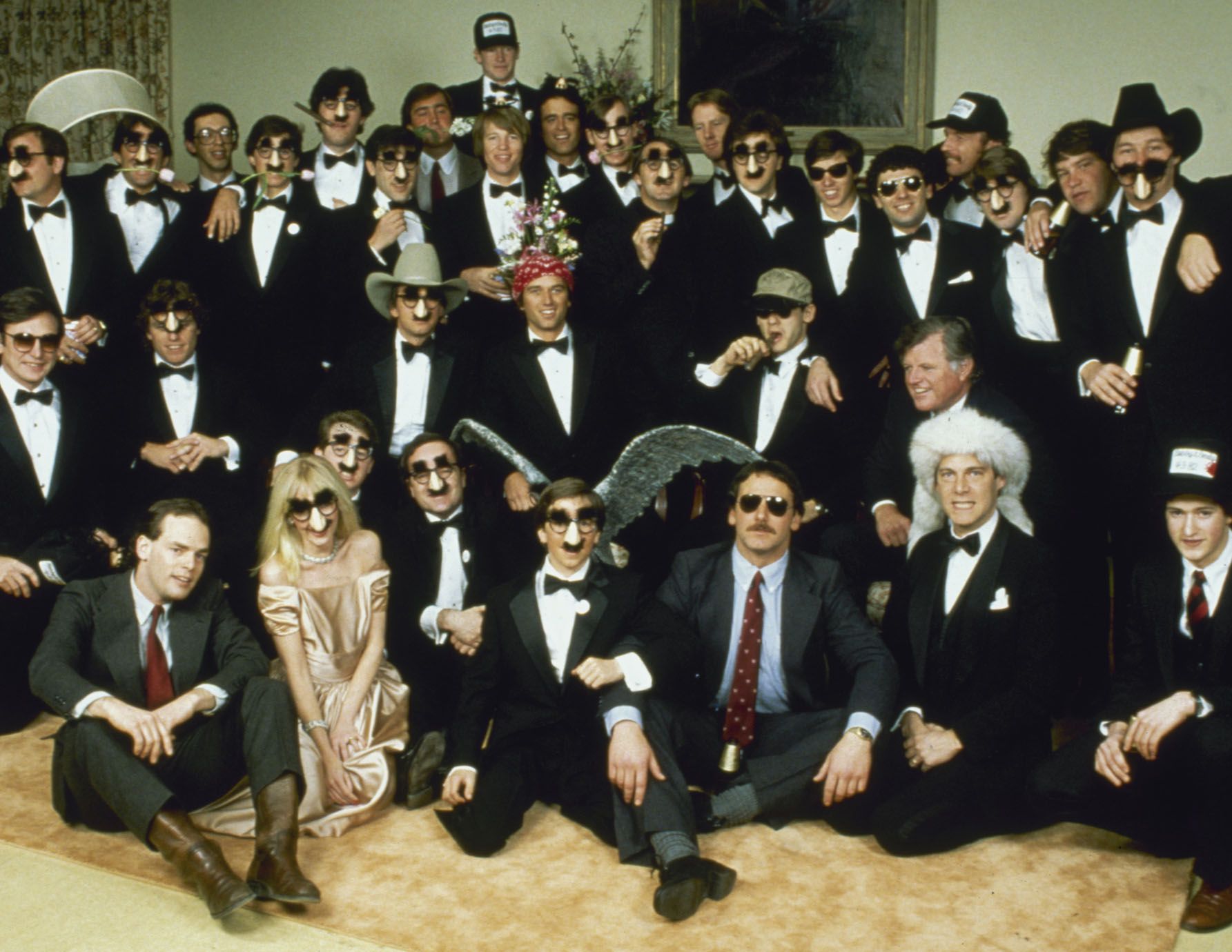
More on Adversarial Images, this time at The Verge, April 12, 2017...

Another Way To Fool The Facial Recognition Algos
Thanks (I think, as long as I don't have nightmares) to a reader.
A quick refresher:...
And finally, the essential "Machine Learning and the Importance of 'Cat Face'".