Our study of 25 years of artificial-intelligence research suggests the era of deep learning is coming to an end.
Almost everything you hear about artificial intelligence today is thanks to deep learning. This category of algorithms works by using statistics to find patterns in data, and it has proved immensely powerful in mimicking human skills such as our ability to see and hear. To a very narrow extent, it can even emulate our ability to reason. These capabilities power Google’s search, Facebook’s news feed, and Netflix’s recommendation engine—and are transforming industries like health care and education.
But though deep learning has singlehandedly thrust AI into the public eye, it represents just a small blip in the history of humanity’s quest to replicate our own intelligence. It’s been at the forefront of that effort for less than 10 years. When you zoom out on the whole history of the field, it’s easy to realize that it could soon be on its way out.
“If somebody had written in 2011 that this was going to be on the front page of newspapers and magazines in a few years, we would’ve been like, ‘Wow, you’re smoking something really strong,’” says Pedro Domingos, a professor of computer science at the University of Washington and author of The Master Algorithm.
The sudden rise and fall of different techniques has characterized AI research for a long time, he says. Every decade has seen a heated competition between different ideas. Then, once in a while, a switch flips, and everyone in the community converges on a specific one.
At MIT Technology Review, we wanted to visualize these fits and starts. So we turned to one of the largest open-source databases of scientific papers, known as the arXiv (pronounced “archive”). We downloaded the abstracts of all 16,625 papers available in the “artificial intelligence” section through November 18, 2018, and tracked the words mentioned through the years to see how the field has evolved.
Through our analysis, we found three major trends: a shift toward machine learning during the late 1990s and early 2000s, a rise in the popularity of neural networks beginning in the early 2010s, and growth in reinforcement learning in the past few years.
There are a couple of caveats. First, the arXiv’s AI section goes back only to 1993, while the term “artificial intelligence” dates to the 1950s, so the database represents just the latest chapters of the field’s history. Second, the papers added to the database each year represent a fraction of the work being done in the field at that moment. Nonetheless, the arXiv offers a great resource for gleaning some of the larger research trends and for seeing the push and pull of different ideas.
A machine-learning paradigm
The biggest shift we found was a transition away from knowledge-based systems by the early 2000s. These computer programs are based on the idea that you can use rules to encode all human knowledge. In their place, researchers turned to machine learning—the parent category of algorithms that includes deep learning.
Among the top 100 words mentioned, those related to knowledge-based systems—like “logic,” “constraint,” and “rule”—saw the greatest decline. Those related to machine learning—like “data,” “network,” and “performance”—saw the highest growth.
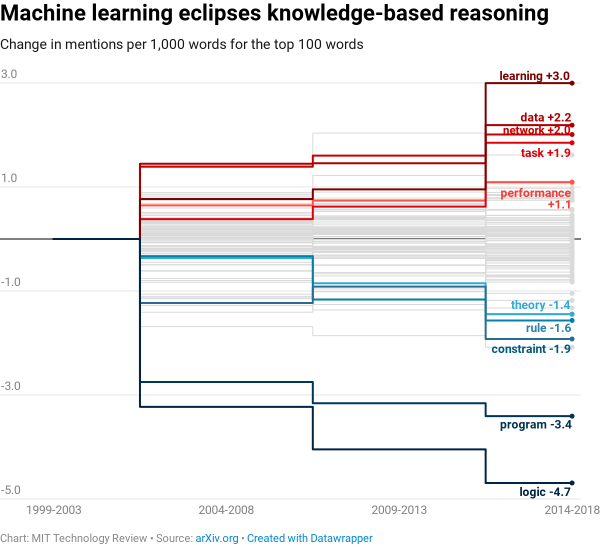
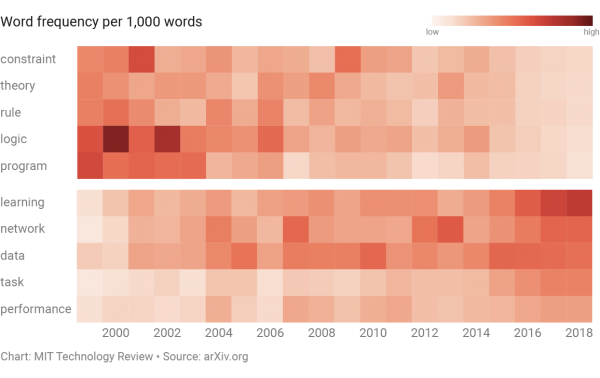
The reason for this sea change is rather simple. In the ’80s, knowledge-based systems amassed a popular following thanks to the excitement surrounding ambitious projects that were attempting to re-create common sense within machines. But as those projects unfolded, researchers hit a major problem: there were simply too many rules that needed to be encoded for a system to do anything useful. This jacked up costs and significantly slowed ongoing efforts.Coincidentally we visited Professor Domingos last Sunday in ""The Master Algorithm Is Going To Change Life As We Know It""
Machine learning became an answer to that problem. Instead of requiring people to manually encode hundreds of thousands of rules, this approach programs machines to extract those rules automatically from a pile of data. Just like that, the field abandoned knowledge-based systems and turned to refining machine learning....MUCH MORE