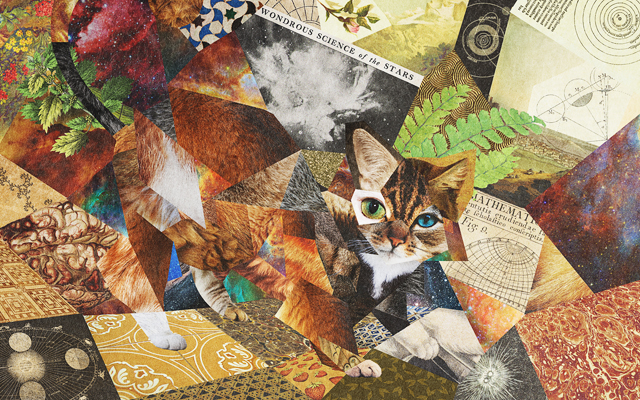
There may be a universal logic to how physicists, computers and brains
tease out important features from among other irrelevant bits of data.
When in 2012 a computer learned to recognize cats in YouTube videos and just last month another correctly captioned a photo of “a group of young people playing a game of Frisbee,” artificial intelligence researchers hailed yet more triumphs in “deep learning,” the wildly successful set of algorithms loosely modeled on the way brains grow sensitive to features of the real world simply through exposure.Previously:
Using the latest deep-learning protocols, computer models consisting of networks of artificial neurons are becoming increasingly adept at image, speech and pattern recognition — core technologies in robotic personal assistants, complex data analysis and self-driving cars. But for all their progress training computers to pick out salient features from other, irrelevant bits of data, researchers have never fully understood why the algorithms or biological learning work.
Now, two physicists have shown that one form of deep learning works exactly like one of the most important and ubiquitous mathematical techniques in physics, a procedure for calculating the large-scale behavior of physical systems such as elementary particles, fluids and the cosmos.
The new work, completed by Pankaj Mehta of Boston University and David Schwab of Northwestern University, demonstrates that a statistical technique called “renormalization,” which allows physicists to accurately describe systems without knowing the exact state of all their component parts, also enables the artificial neural networks to categorize data as, say, “a cat” regardless of its color, size or posture in a given video.
“They actually wrote down on paper, with exact proofs, something that people only dreamed existed,” said Ilya Nemenman, a biophysicist at Emory University. “Extracting relevant features in the context of statistical physics and extracting relevant features in the context of deep learning are not just similar words, they are one and the same.”
As for our own remarkable knack for spotting a cat in the bushes, a familiar face in a crowd or indeed any object amid the swirl of color, texture and sound that surrounds us, strong similarities between deep learning and biological learning suggest that the brain may also employ a form of renormalization to make sense of the world....MORE
"Machine-Learning Maestro Michael Jordan on the Delusions of Big Data and Other Huge Engineering Efforts"
'Deep Learning' as Applied to Investing
Deep Learning is VC Worthy
"Why Is Machine Learning (CS 229) The Most Popular Course At Stanford?"
Zuckerberg, Musk Invest in Machine Learning Artificial-Intelligence Company, Vicarious
Google's Plan To Make Your Brain Irrelevant (GOOG; EVIL;)
MIT's Technology Review: "10 technologies we think most likely to change the world"
"As Machines Get Smarter, Evidence They Learn Like Us"
Researcher Dreams Up Machines That Learn Without Humans